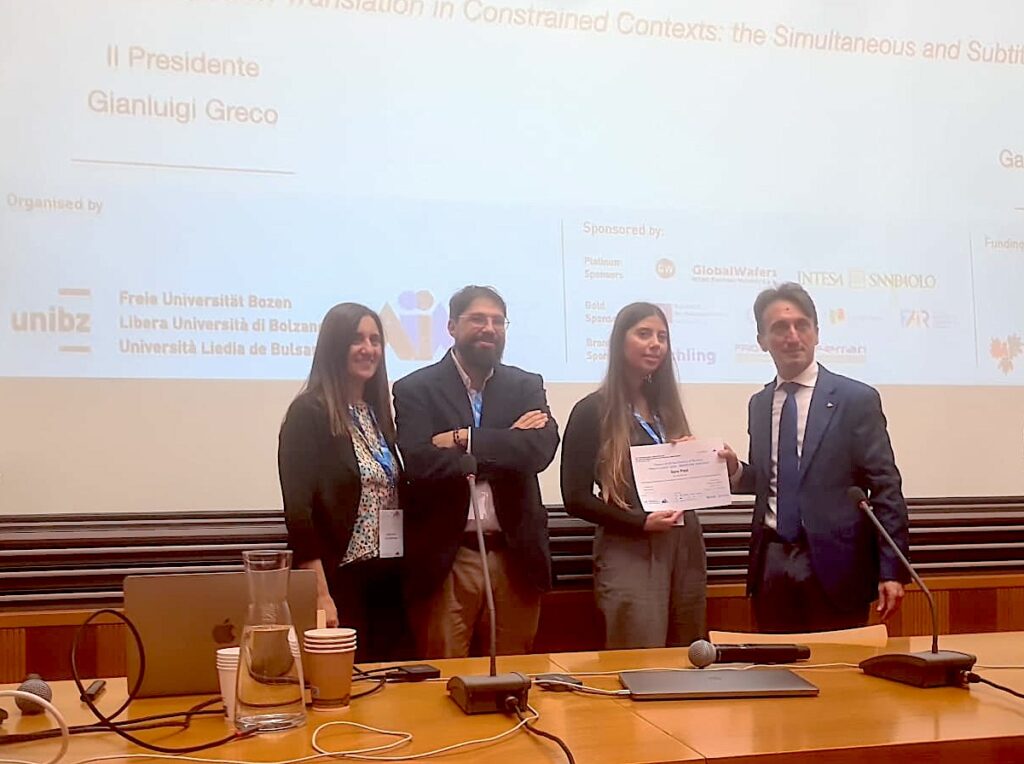
We are glad to announce that our Sara Papi received the Special Mention for the Best PhD Thesis Award assigned by AIxIA 2024!
The details of the thesis are the following:
- Title: Direct Speech Translation in Constrained Contexts: the Simultaneous and Subtitling Scenarios
- Link: https://iris.unitn.it/handle/11572/407270
- Abstract: This PhD thesis summarizes the results of a three-year comprehensive investigation into the dynamic domain of speech translation (ST), with a specific emphasis on application scenarios requiring adherence to the additional constraints posed by simultaneous speech translation and automatic subtitling. These additional constraints, revolving around aspects such as latency and on-screen spatio-temporal conformity, add layers of complexity and thereby complicate the inherent challenges of ST. I started my exploration with a novel paradigm, direct speech translation, which was in its early stages during the beginning of my journey. Along this direction, in the pursuit of advancing simultaneous ST (SimulST), my research challenged the conventional approach of creating task-specific direct architectures. Instead, the focus was on leveraging the intrinsic knowledge acquired by offline-trained direct ST models for simultaneous inference. A pivotal contribution of this endeavor was the finding that offline-trained ST systems can not only compete with but potentially surpass the quality and latency of those specifically trained for simultaneous scenarios. An important subsequent step has been taken by leveraging cross-attention information extracted from an offline direct ST model for SimulST, demonstrating its potential to deliver high-quality, low-latency translations with minimal computational costs and thus achieve an optimal balance between translation quality and latency. The exploration of automatic subtitling delved into the complexities of spatio-temporal constraints, highlighting the interplay between translation quality, text length, and display duration. The recognition of the importance of prosody and speech cues shaped the development of direct architectures for the task. Relevant findings include the effectiveness of a multimodal segmenter, leveraging both audio and textual cues for optimal segmentation into subtitles. Furthermore, my research showcased the capability of direct ST models to generate complete subtitles, offering translations appropriately segmented with corresponding timestamps, and demonstrating competitive performance against existing cascaded production tools. In conclusion, the insights gleaned in this PhD from both fields mark substantial technological progress, which I believe will set the stage for the wide adoption of direct ST systems in the two challenging domains of simultaneous ST and automatic subtitling.
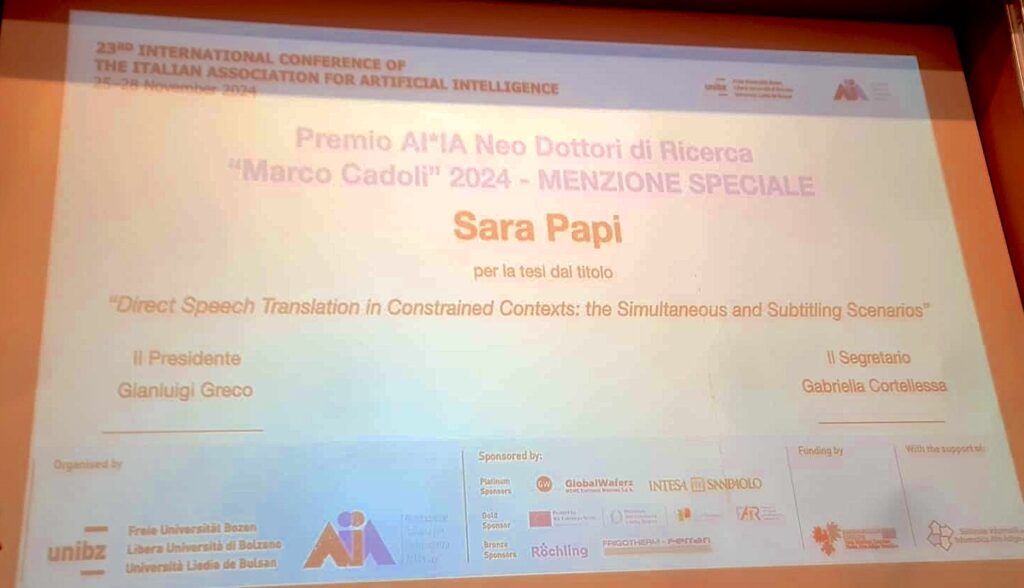